国際ジャーナル 「IEEE Access」に1件採択!
2023年7月26日 最終更新日時 : 2023年7月26日 report
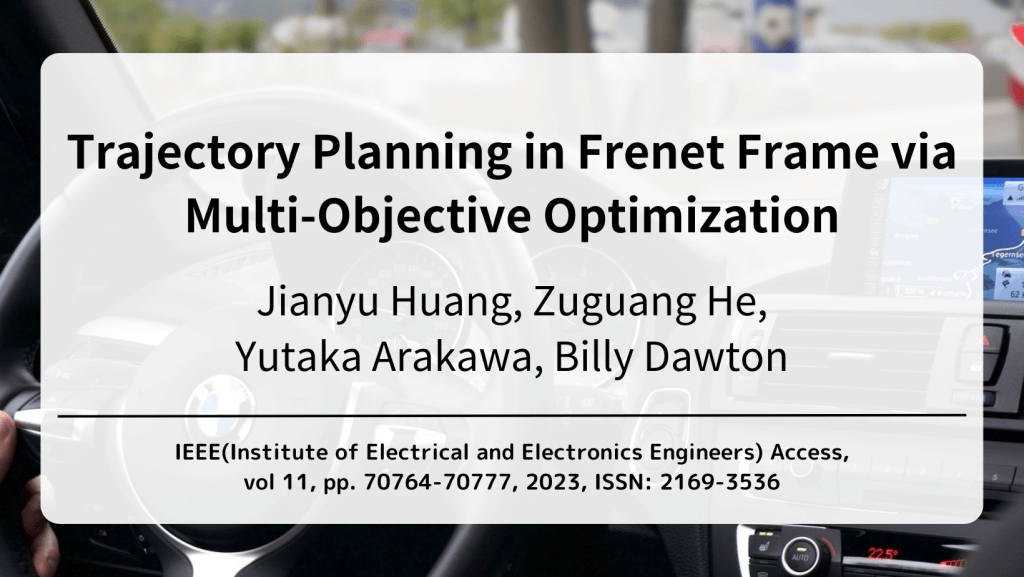
国際ジャーナル「IEEE Access」に下記の論文が採択されました.
Jianyu Huang, Zuguang He, Yutaka Arakawa, Billy Dawton
Trajectory Planning in Frenet Frame via Multi-Objective Optimization Journal Article
In: IEEE Access, vol. 11, pp. 70764-70777, 2023, ISSN: 2169-3536.
@article{access2023-huang,
title = {Trajectory Planning in Frenet Frame via Multi-Objective Optimization},
author = {Jianyu Huang, Zuguang He, Yutaka Arakawa, Billy Dawton},
url = {https://app.ait.kyushu-u.ac.jp/wp-content/uploads/2023/07/Trajectory_Planning_in_Frenet_Frame_via_Multi-Objective_Optimization.pdf},
doi = {10.1109/ACCESS.2023.3294713},
issn = {2169-3536},
year = {2023},
date = {2023-07-12},
urldate = {2023-07-12},
journal = {IEEE Access},
volume = {11},
pages = {70764-70777},
abstract = {Autonomous vehicles are an essential tool for promoting the development of intelligent transportation systems (ITS) and can effectively reduce traffic accidents caused by human errors. As an important part of the automatic driving software system, path planning is responsible for generating the motion trajectory of the vehicle, which is the primary factor determining driving quality. However, solution space construction and optimization problem formulation remain challenging research areas in the field of path planning. In this paper, we propose a multi-objective optimization algorithm for static obstacle avoidance to improve the comfort, safety and anti-deviation of the planned trajectory. We decouple the lateral and longitudinal motion of the vehicle using the Frenet frame and discretize the driving state space to generate target states of the vehicle. Based on the initial and target states, we generate a set of lateral and longitudinal motion trajectories using quintic and quartic polynomials, respectively. In addition, we design a cost function that comprehensively considers the comfort, safety, and deviation distance of the road center line by combining an acceleration check, curvature check, and collision check. As part of the cost function, we propose a novel method to quantify the safety of candidate trajectories considering the size of obstacles. The experimental results show that the proposed algorithm can quantize the safety of candidate paths and improve comfort 13.47%, 32.19%, 59.36% and 18.60% on a straight road, curvy road, intersection and U-shaped road, respectively. Furthermore, the algorithm can improve anti-deviation by 63.72%, 13.86%, 44.36%, and 45.56% on a straight road, curvy road, intersection and U-shaped road, respectively.},
keywords = {},
pubstate = {published},
tppubtype = {article}
}
Autonomous vehicles are an essential tool for promoting the development of intelligent transportation systems (ITS) and can effectively reduce traffic accidents caused by human errors. As an important part of the automatic driving software system, path planning is responsible for generating the motion trajectory of the vehicle, which is the primary factor determining driving quality. However, solution space construction and optimization problem formulation remain challenging research areas in the field of path planning. In this paper, we propose a multi-objective optimization algorithm for static obstacle avoidance to improve the comfort, safety and anti-deviation of the planned trajectory. We decouple the lateral and longitudinal motion of the vehicle using the Frenet frame and discretize the driving state space to generate target states of the vehicle. Based on the initial and target states, we generate a set of lateral and longitudinal motion trajectories using quintic and quartic polynomials, respectively. In addition, we design a cost function that comprehensively considers the comfort, safety, and deviation distance of the road center line by combining an acceleration check, curvature check, and collision check. As part of the cost function, we propose a novel method to quantify the safety of candidate trajectories considering the size of obstacles. The experimental results show that the proposed algorithm can quantize the safety of candidate paths and improve comfort 13.47%, 32.19%, 59.36% and 18.60% on a straight road, curvy road, intersection and U-shaped road, respectively. Furthermore, the algorithm can improve anti-deviation by 63.72%, 13.86%, 44.36%, and 45.56% on a straight road, curvy road, intersection and U-shaped road, respectively.