IEEE Accessに2件採択
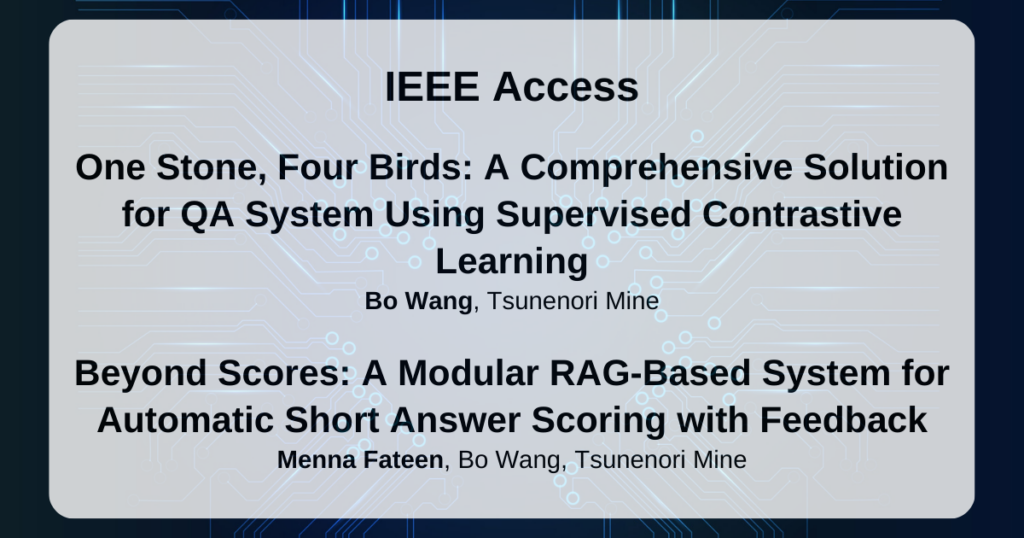
IEEE Accessに2件の論文が採択されました。
Menna Fateen, Bo Wang, Tsunenori Mine Beyond Scores: A Modular RAG-Based System for Automatic Short Answer Scoring with Feedback Journal Article In: IEEE Access, vol. 12, pp. 1 - 1, 2024. Bo Wang, Tsunenori Mine One Stone, Four Birds: A Comprehensive Solution for QA System Using Supervised Contrastive Learning Journal Article In: IEEE Access, vol. 12, pp. 148488 - 148501, 2024.@article{mennna2024access,
title = {Beyond Scores: A Modular RAG-Based System for Automatic Short Answer Scoring with Feedback},
author = {Menna Fateen, Bo Wang, Tsunenori Mine},
year = {2024},
date = {2024-11-29},
urldate = {2024-11-29},
journal = {IEEE Access},
volume = {12},
pages = {1 - 1},
abstract = {Automatic short answer scoring (ASAS) helps reduce the grading burden on educators but
often lacks detailed, explainable feedback. Existing methods in ASAS with feedback (ASAS-F) rely on fine-tuning language models with limited datasets, which is resource-intensive and struggles to generalize across contexts. Recent approaches using large language models (LLMs) have focused on scoring without extensive fine-tuning. However, they often rely heavily on prompt engineering and either fail to generate elaborated feedback or do not adequately evaluate it. n this paper, we propose a modular retrieval augmented generation (RAG) based ASAS-F system, utilizing RAG as a few-shot selection method to score answers
and generate feedback in zero-shot and few-shot learning scenarios. We design our system to be adaptable without extensive prompt engineering using an automatic prompt generation framework. Results show an improvement in scoring accuracy by 9% on unseen questions compared to fine-tuning, offering a scalable and cost-effective solution.},
keywords = {},
pubstate = {published},
tppubtype = {article}
}
often lacks detailed, explainable feedback. Existing methods in ASAS with feedback (ASAS-F) rely on fine-tuning language models with limited datasets, which is resource-intensive and struggles to generalize across contexts. Recent approaches using large language models (LLMs) have focused on scoring without extensive fine-tuning. However, they often rely heavily on prompt engineering and either fail to generate elaborated feedback or do not adequately evaluate it. n this paper, we propose a modular retrieval augmented generation (RAG) based ASAS-F system, utilizing RAG as a few-shot selection method to score answers
and generate feedback in zero-shot and few-shot learning scenarios. We design our system to be adaptable without extensive prompt engineering using an automatic prompt generation framework. Results show an improvement in scoring accuracy by 9% on unseen questions compared to fine-tuning, offering a scalable and cost-effective solution.@article{wang2024access,
title = {One Stone, Four Birds: A Comprehensive Solution for QA System Using Supervised Contrastive Learning},
author = {Bo Wang, Tsunenori Mine},
year = {2024},
date = {2024-09-27},
urldate = {2024-09-27},
journal = {IEEE Access},
volume = {12},
pages = {148488 - 148501},
abstract = {This paper presents a novel and comprehensive solution to enhance both the robustness and efficiency of question answering (QA) systems through supervised contrastive learning (SCL). Training a high-performance QA system has become straightforward with pre-trained language models, requiring only a small amount of data and simple fine-tuning. However, despite recent advances, existing QA systems still exhibit significant deficiencies in functionality and training efficiency. We address the functionality issue by defining four key tasks: user input intent classification, out-of-domain input detection, new intent discovery, and continual learning. We then leverage a unified SCL-based representation learning method to efficiently build an intra-class compact and inter-class scattered feature space, facilitating both known intent classification and unknown intent detection and discovery. Consequently, with minimal additional tuning on downstream tasks, our approach significantly improves model efficiency and achieves new state-of-the-art performance across all tasks.},
keywords = {},
pubstate = {published},
tppubtype = {article}
}